NOx Emission Modelling and Control for Fossil-fuel Power Generation Plants
Researchers : Dr. Kang Li, Dr Jian-Xun Peng and Dr Stephen Thompson
Introduction
In the past decade, atmospheric pollution has become a major global concern due to ever increasing demand on energy and raw material consumptions in both developed and developing countries. The major contributors to atmospheric pollution are mainly from fossil-fuel power stations (see figure 1) and transportation vehicles. Ever increasing demand on energy has made the problem even more challenging. The principal pollutants from these energy systems may include sulphur dioxide (SO2), nitrogen oxides (NOx), carbon dioxide (CO2) and particulates (soot, flyash). Among these emissions, no practical methods exist for reducing CO2 or NOx significantly, leading to increased research into these two important areas. This project focuses on the modelling, prediction and control of NOx emissions from fossil-fuel power generation plants.
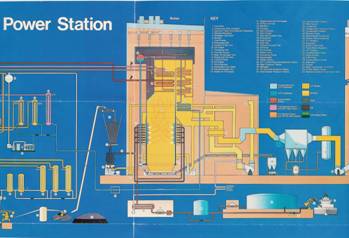
Fig 1. Coal-fired power plants
Methods and results
The earliest research into NOx formation mechanism can be traced back to 1943 when Zeldovich first postulated the thermal NO formation mechanism. However, extensive research started following the discussions on acidification in the eighties. These would include papers on NOx formation, modelling and control. Technologies for NOx emission reduction may be classified into two groups:
- primary or combustion modification-based technologies, and
- secondary or flue-gas treatment-based technologies
The early techniques used in this project include some ‘black-box’ linear and nonlinear regression (ARX and NARX) models, and artificial neural networks for modelling and control purpose. Each of these models has both advantages and disadvantages, mainly on their model complexity and generalisation.
However, power plant NOx emission modelling for real-time operation and control presents some unique problems.
- The fundamental equations for NOx formation cannot be directly applied as many of the variables are either not measurable online or cannot be reliably measured. Therefore, the model structure is not readily available, thus excluding non-linear system parameter estimation techniques.
- Information such as the steady-state relationships between input and output is not readily available. This causes problems in the construction of a lumped system model, or a Wiener, Hammerstein, or Wiener-Hammerstein model of the process.
- During normal plant operation, safety and production considerations mean that test signals are highly restricted in terms of magnitudes and duration.
To tackle these problems, this project then further developed a novel 'grey-box' approach, which uses equations relating the NOx formation mechanism in order to extract some fundamental functions which are referred to FEs (fundamental elements). An algorithm is then used to construct the 'best' time series NOx emission model by selecting the most significant of the functions. The developed model has been used to predict NOx emissions from two different power plants for over a month.
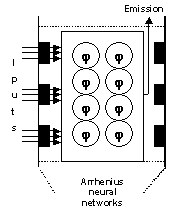
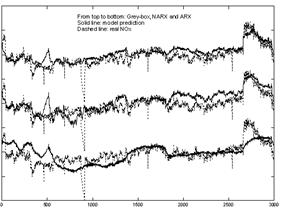
Fig 2. Neural mesh estimation of pollutant
emissions in TPPs using Arrhenius function
The industry has mature experience on modelling and control of power plants using conventional neural networks, and CFD method has been well established as the best tool for plant design by simulating the combustion process in the furnace to estimate pollutant emissions from the TPPs. The first model type lacks transparency, the second method is however very expensive to implement and is also time consuming. The proposal of this above transparent neural network paradigm offers a new prospect in real-time power plant emission modelling and control with improved performance and model transparency.
Moreover, a software package has been developed for modelling NOx emissions (see Figure 3) using genetic algorithm.
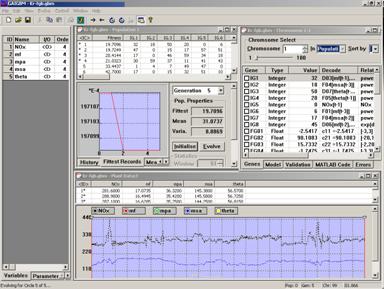
Fig 3. NOx emission modelling software
Acknowledgements
This work has been partially supported by the Engineering and Physical Sciences Research Council (EPSRC) for funding this project (GR/S85191/01), The British Coal Utilization Research Association.
Keynote speech
- K. Li, “General Introduction to Nonlinear System Modeling & Identification”, 18th International Conference and Fair for Measurement Instrumentation and Automation, Everbright Exhibition Centre, Shanghai, 19 September, 2007.
Invited lectures
- The University of Sheffield , “Construction and training of generalised single hidden-layer neural networks”, 31 Oct, 2007, Sheffield, UK.
- University of Iowa . “Advanced generic algorithm for nonlinear system modelling and optimisation”, 3 July, 2007, Iowa City, USA.
- Polytechnic University of Bari, “Intelligent System Modelling and Control with application to some environmental issues”, March 27-31, 2006, Taranto, Italy.
- Institute of Intelligent Machines, Chinese Academic of Sciences, “Eng-genes: a genetic modelling approach for nonlinear dynamic systems”, April 08, 2005, Hefei, China.
- Shangdong University , “Eng-genes: a genetic modelling approach for nonlinear dynamic systems”, April 12, 2005, Shandong, China.
- University of Glamorgan , “A grey-box approach for nonlinear dynamic system modelling and identification”, Nov. 19, 2004, UK.
- Shanghai Jiaotong University , ‘Nonlinear dynamic system modelling and identification using grey-box methods’, June 21, 2004, Shanghai, China.
- Shanghai University , ‘‘Nonlinear dynamic system modelling and identification using grey-box methods’’, June 24, 2004.
Publications
- K. Li, J. Peng, S. Thompson, “Genetic neural Modeling of pollutant Emissions from thermal power plants”, Dynamics of Continuous, Discrete and Impulsive Systems Series B: Applications & Algorithms, Vol. 14 (S1), pp 48-56, 2007.
- K. Li, S. Thompson, J. Peng, “Modelling and prediction of NOx emission in a coal-fired power generation plant", Control Engineering Practice, Vol. 12, 707-723, 2004.
- K. Li, S. Thompson, “NOx emission models suitable for operation and control of power generation boilers", Clean Air: International Journal on Energy for a Clean Environment, Vol 5, 89-105, Begell House, 2004.
- K. Li, S. Thompson, P.A. Wieringa, J. Peng, G. Duan, "Neural networks and genetic algorithms can support human supervisory control to reduce fossil fuel power plant emissions". Cognition, Technology & Work, Springer-Verlag, ISSN: 1435-5558, Vol. 5, No. 2, pp:107-126, June 2003.
- S. Thompson, and K. Li, “Modelling of NOx emissions in coal-fired plants”, in Thermal Power Plant Simulation and Control, Flynn(ed.), Power and Energy Series 43, IEE Press, pp: 243-268, ISBN: 085296496, 2003.
- J. Peng, K. Li, D.S. Huang. “A Hybrid forward Algorithm for RBF neural Network construction”. IEEE Transactions on Neural Networks, Vol 17, No. 6, pp 1439-1451, 2006.
- W. Spinelli, L. Piroddi, K. Li, "Nonlinear modeling of NOx emission in a coal-fired power generation plant", 43rd IEEE International Conference on Decision and Control, Paradise Island, Bahamas, December 14-17, 2004.
- J. Peng, S. Thompson, K. Li, “A Hybrid Genetic Algorithm for NOx Emission Modelling in Power Generation Plants”, 15th IFAC World Congress on Automatic Control, Barcelona, CDROM, July 2002.
- J. Peng, K. Li, S. Thompson, “GA based software for power generation plant NOx emission modelling”, 6th International Conference on Technologies and Combustion for A Clean Environment, Vol. 2, 881-887, Porto, Portugal, 2001.
- S. Thompson, K. Li, “A MLP Prediction Model for Power Plant NOx emission”, IFAC Workshop on Algorithms and Architectures for Real-Time Control (AARTC2000), Spain, 5-17 May, 2000, Eds V. Hernández and G. W. Irwin, pp 108-114.
- K. Li, S. Thompson, “Cascading neural network and its application to modeling power plant pollutant emission”, The Third World Congress on Intelligent Control and Intelligent Automation (WCICA'2000), Vol. 2, Hefei, China, 2000, 992-997.
- K. Li, S. Thompson, “NOx Emission Modeling and Prediction of a 200-MWe Power Generation Boiler Using Multilayer Perceptron Neural Networks”, 31st Annual North American Power Symposium, San Luis Obispo, USA, 1999, 218-224.
- N. Li, K. Li, S. Thompson, "Employing a new type of neural network to optimise power plant air/fuel ratio", 14th IFAC World Congress, Vol. O, pp. 333-338, Beijing, China, 1999.