An Integrated System of inferential measurement and control of polymer extrusion for self-tuning optimisation and response to disturbances
Final Report
EPSRC Grant EP/F021070/1
Prof Kang Li+, Dr Peter Martin*
+School of Electronics, Electrical Engineering & Computer Science, Queen’s University Belfast
*School of Mechanical & Aerospace Engineering, Queen’s University Belfast
Click here to a pdf copy of the complete report.
Summary: The polymer industry makes a major contribution to the UK economy, and extrusion is a fundamental polymer processing stage. However, considerable waste of both energy and raw materials can occur during lengthy change-over and start-up periods, and conservative operating conditions make this situation worse. Furthermore, the extrusion process is highly complex, and incomplete melting of the polymer may occur at inappropriate processing conditions that give rise to poor mixing and localised hot and cold spots, which in turn lead to pulsations in the throughput. As the key melt indicator, viscosity is difficult to monitor online, and as a result of this and the process characteristics described it is difficult to control the quality of an extrudate material and hence the final product. The aims of this research were to advance the state of the art in monitoring and control of polymer extrusion so as to reduce set-up times, waste and energy consumption and improve product quality. Considerable achievements have been made: 1) We have investigated the pressure model incorporating changes in the machine/die and material properties. 2) We have developed a model identification procedure based on grey-box modelling with a genetic algorithm for viscosity modelling, and further improved a soft sensor approach with a feedback structure to enable the online adaptability of the viscosity model in response to modelling errors and disturbances, hence producing a reliable estimate of viscosity. 3) We have developed a model free fuzzy controller to minimize the variation in melt pressure and temperature, thus reducing the variation of viscosity. 4) We have developed torque signal acquisition methods and investigated an inferential monitoring approach to the screw load torque signal in an extruder. 5) We have employed a thermocouple mesh technique developed by the University of Bradford to measure the die melt temperature profile of a single screw extruder and developed a nonlinear model to predict the die melt temperature profile from readily measured process parameters. The model was then used to identify the effects of individual processing parameters on the die melt flow homogeneity and to further identify the optimal process settings to minimise melt temperature variance across the melt flow. 6) We have explored energy constraints and developed a strategy for responding to process instabilities, then investigated a model based fuzzy control approach to maintain the die melt temperature variance across the melt flow while achieving the desired average die melt temperature. We have further developed a fuzzy controller for the control of the polymer melt quality including viscosity. 7) We have developed a novel nonlinear multivariate statistical approach based on neural networks for fault detection in polymer processing. 8) We have further proposed novel algorithms for the construction of neural networks and fuzzy neural systems, and nonlinear system modelling and classification. These methods have also been applied to the modelling and control of injection stretch blow moulding. All these new developments have taken into account in the industrial environment, and close collaboration with other leading research groups in the UK and with local industrial companies has enabled the direct application of the techniques developed to maximize the impact. Theoretical results have been published in high quality peer reviewed journals, such as Polymer Engineering and Science, Control Engineering Practice, IEEE Transactions on Fuzzy Systems, and Journal of Process Control, and in leading conferences. The publication record (11 journal papers and 16 conference papers, together with 1 PhD thesis , 1 MEng final year project report, and a few more in the pipeline), the award of an international conference prize, and a number of invited seminars and presentations have very effectively demonstrated the high quality of the research outputs.
Beneficiary: This project has significant benefit, firstly, to the UK polymer industry. Polymers, because of their range of properties and ease of processing into complex shapes, are among the most important materials available to us today and the polymer industry makes a major contribution to the UK economy (18 billion per year). The sector is experiencing strong growth due to developments in materials science and the increasing application of high end polymers in diverse industrial sectors; thus resulting in demands for progressively more stringent quality specifications and customisation of products. Extrusion is fundamental for the processing of most polymer materials is required for the production of the vast majority of polymer and composite products. Unfortunately, it is unpredictable and highly prone to disturbances. Historically, long set-up times have been required to identify suitable operating conditions to achieve satisfactory product quality. Considerable energy and raw material is wasted during change-over and start-up periods which can account for up to 10% of the processing time within a typical SME. Waste material from the extrusion industry in the UK is of the order of thousands of tonnes per year (although some can be recycled at additional energy cost) and it is estimated that up to 15% of the energy consumption in the sector (in excess of 255,000MWh per annum) is expended in non-productive or inefficient processing. With increasing energy and raw material costs, and greater awareness of carbon emissions and depleting resources, this results in serious economic concerns for the industry. Greater flexibility in process control are further demanded through trends towards co-extrusion (requiring machines to handle broad material and melt throughput ranges), non-homogeneous starting materials (e.g. recyclate), and an ever-widening range of materials available. This project represents significant advancement over previous attempts at extrusion control, to achieve robust and adaptable control enabling a much more holistic approach to the optimisation of settings, disturbance rejection and self-tuning ability than previously attempted. This is the key to addressing the poor energy efficiency and high volume of waste currently threatening the competitiveness of the UK polymer industry. Furthermore, the developments in process modelling and monitoring of key parameters for process optimisation and control provide an ideal platform for achieving greater success in emerging complex processing applications. Developments in polymer synthesis, blending and nanocomposites highlight the potential for vastly superior materials in future. However, significant progress in understanding and controlling the processing of such materials is required if such advances are to become a commercial reality. Also, as extrusion is fundamental in injection and blow moulding and also in the food industry, the potential impact is extremely wide. The development of novel techniques for semi-physical modelling of complex systems has wider interests beyond polymer processing. During the project period, the team established close collaboration with the IRC centre in Polymer Science and Technology at the University of Bradford, a leading research group in the UK, and collaborated closely with local polymer companies, such as Greiner Packaging Ltd and Cherry Pipes Ltd. Such collaborations have not only enabled the use of a range of facilities and novel measurement technologies to advance the monitoring, modelling and control of the polymer extrusion process, but also helped to ensure the industrial applicability of the newly developed methods and algorithms for improving product quality and reducing energy consumption and material waste.
Objectives:This project aimed to advance the state of the art in monitoring and control of polymer extrusion processing to reduce set-up times, waste and energy consumption and improve product quality. The principal aim was to develop an innovative, integrated system of measurement, modelling, data analysis and algorithms for on-line tracking and optimisation of the key performance targets of the process and to recognise and respond appropriately to disturbances. The system is able to be self-tuning to different materials. The secondary aim of this research was to highlight techniques in process modelling and on-line measurement and analysis which could form a basis for further research in the processing of other materials. The individual objectives of this research included: 1) an integrated framework has been developed for improved performance of extrusion processes through advances in process modelling, inferential measurement and a more intelligent approach to control of the process; 2) novel modelling techniques have been developed which are capable of capturing the complex nature of interactions between process inputs and outputs of interest based on industrial application environment. This has included the development of mechanisms for on-line updating of models to reflect changes in material properties and processing conditions; 3) inferential measurement techniques have been developed for tracking melt viscosity and identification of process instabilities; 4) An intelligent control strategy has been proposed to achieve the required product quality at maximum efficiency and respond appropriately to process faults / material degradation, and melting instabilities and material changes.
1. Background
The operation and control of the extrusion process presents several key challenges. Firstly, the selection of appropriate operating conditions for each material to obtain a desired extrudate quality is a complex task. Historically, long set-up times have been required if the extrusion condition changes, e.g. the extrudate material, throughput, or extruder geometry varies. This leads to considerable waste of energy and raw material during change-over and start-up periods which can account for up to 10% of processing time for a typical SME. Furthermore, non-optimum operating conditions make the waste of energy and material even much worse. Secondly, the extrusion process is highly nonlinear with varying time delays, and incomplete melting of the polymer occurs at inappropriate processing conditions giving rise to poor mixing of localised hotspots and cool areas, leading to pulsations in the throughput. Further, as a key melt indicator, viscosity is difficult to monitor online. These features makes it difficult to control the quality of an extrudate material and hence the final product. Real-time monitoring, control and optimization of the extrusion process are therefore desirable to achieve high quality polymer products with reduced set-up time and improved energy efficiency.
Varying degrees of success have been achieved in the area of modelling and control of the extrusion process in the last few decades, often by manipulating the barrel temperature and screw speed settings using empirical modelling techniques. Control of melt homogeneity and throughput has traditionally been attempted by joint regulation of melt pressure and melt temperature. The majority of schemes have concentrated on linear lumped-parameter techniques and PID approaches to modelling and control of these variables. Such linear techniques have shown the benefit of reducing the fluctuation in melt pressure and temperature at fixed operating conditions. But as the process is highly non-linear with varying time delays, they are not suitable for wider operating ranges, especially when facing the challenge of the increasing use of recycled materials and more stringent quality and energy requirements. In particular, a fundamental issue is that the melt temperature and pressure are poor performance indicators, as they are specific to fixed material properties and machine set-up and provide no insight to functional product properties. Viscosity itself is largely recognised as the most relevant indicator of melt quality as it dictates the aesthetic and dimensional properties of the melt and can indicate a change in molecular structure and/or orientation relating to functional properties. Control of the melt viscosity is paramount in achieving stable die flow and in facilitating the required draw-down of the melt in subsequent haul-off or film-blowing processes. Lack of suitable instrumentation has hampered development of viscosity control schemes although existing research [1] has attempted a number of different strategies to control viscosity based on in-line rheometer measurements. Screw speed was used as the only manipulating variable, and both model generation and testing of controller performance were limited to singular step changes at a fixed operating point, which are not realistic to achieve in practical operation.To overcome these shortcomings, within this project we have: (1) carried out fundamental research on the modelling of relationships between the process settings and key process output variables, including die temperature profile, pressure, and in particular the viscosity, which can be applied to a wide range of machines and to the changes in feed materials; (2) developed novel techniques for online identification of process disturbances, investigated melting instabilities in the process, and proposed new optimization and control strategies to minimise off-specification product and down time.
This research has been closely linked with several other projects including an EU FP7 project ‘Ultravisc’ and an on-going EPSRC project on ‘Thermal management in polymer processing’ (EP/G059489/1). The former is concerned with controlling melt viscosity in the processing of recycled polymers using ultrasonically assisted viscosity modulation as well as attempting to develop an ultrasound-assisted filtration system, whereas the latter is concerned with controlling the energy use and efficiency of the polymer industry.
2. Aims and Objectives
The original aims of the research were to advance the state of the art in monitoring and control of the polymer extrusion process to reduce set-up times, waste and energy consumption and improve product quality.
- The principal aim was to develop an innovative, integrated system of measurement, modelling, data analysis and algorithms for on-line tracking and optimisation of the key performance targets of the process and to recognise and respond appropriately to disturbances. The system is required to be self-tuning to different materials.
- As a secondary aim, this research would highlight techniques in process modelling and on-line measurement and analysis which could form a basis for further research in processing of complex, high performance materials, particularly through reactive extrusion and dispersion of nanoscale fillers.
The original individual objectives of this research included:
- To develop an integrated framework for improved performance of extrusion processes through advances in process modelling, inferential measurement and a more intelligent approach to control of the process.
- To investigate novel modelling techniques capable of capturing the complex nature of interactions between process inputs and outputs of interest on industrial scale extruders. This includes the development of mechanisms for on-line updating of models to reflect changes in material properties and processing conditions.
- To develop inferential measurement techniques for tracking melt viscosity and identification of process instabilities.
- To develop a control strategy to achieve the required product quality at maximum efficiency and respond appropriately to process faults – material degradation, solids conveying and melting instabilities and material changes.
- To verify developments in an industrial setting.
3. The methodology and key advances
The original objectives of the project were specified in a number of tasks and sub-tasks. These are repeated here along with our contributions to each one. The outputs from the project (see section 6 below) include 11 refereed journal papers, 16 conference papers, and a PhD thesis and a MEng final year project report.
Task A. Tracking of viscosity
Task A.1 Viscosity monitoring
To obtain real-time inferential estimation of the melt viscosity, pressure drop along the die is required. We have investigated the pressure model incorporating changes in machine/die and material properties, and carried out a number of tests to validate this model. In particular, given the consideration that the die pressure is not always available, to apply the proposed method for industrial applications, the barrel pressure which is available in industrial processes was examined instead of the die pressure in the pressure model. Based on previous research, we have employed a FIR (finite impulse response) model for the pressure instead of the NARX (nonlinear autoregressive with exogeneous input) model, which has the advantage of inherent stability, without the need for feedback but exhibiting good performance. We found that (1) the improved FIR model has less terms in the predefined ‘term pool’, hence leading to a simplified fixed structure; (2) the identified pressure model with a simplified fixed structure can capture the dynamic relationship between the viscosity, screw speed and extruder barrel pressure; (3) the pressure model is affected by the material with a small offset; (4) the pressure model with a simplified fixed structure can be generalized to different machines and dies; (5) only the linear parameters need to be identified for such a model when applied in a different die/machine [C1].
Task A.2 Viscosity modelling
We have reviewed first-principle models, carried out five extrusion trials with five polymer materials and 2 dies and 2 extruders for data generation, and developed a model identification procedure based on grey-box modelling with a genetic algorithm [5,6,7]. From a control point view, it is known that both the barrel temperature and screw speed have significant effect on the quality of the final product and the energy/thermal efficiency of the process. Thus, both barrel temperature and screw speed have been used as input variables to build the viscosity model. It is noted that similar work has been reported in [2] which developed dynamic grey-box models relating melt pressure and melt temperature to screw speed (or feed rate) alone with random binary sequence (or stair) type excitation for twin screw extrusion. In contrast to [3,4], in this project, four input variables including three barrel temperature and screw speed, have been excited simultaneously with a pre-designed Pseudo-random signal (PRS) and hence a wide range of operating conditions has been covered for a single screw extruder. We have found out that (1) a FIR model can be identified for the estimation of the melt viscosity based on the process inputs such as screw speed and temperature; (2) the viscosity model has a simple structure with adaptable linear parameters for different materials [J1].
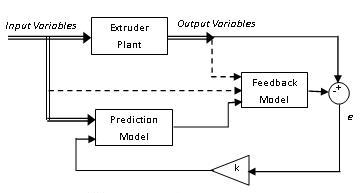
In spite of the effectiveness of the grey-box modeling technique assisted with genetic algorithms, which combines both fundamental first principles and an experimental data-driven approach, the existing methods are inherently open-loop, and therefore suffer from all the problems associated with open-loop systems, i.e. they are intolerant to any changes in the extrudate material, machine geometry, or processing conditions. To tackle this problem, a soft sensor approach with a feedback structure was proposed in previous work [2,4]. The soft sensor structure is based on that of a state observer where an open-loop estimator (viscosity model) is combined with a feedback correction mechanism performed by a pressure model. The novel feedback structure enables the online adaptability of the viscosity model in response to modelling errors and disturbances, hence producing a reliable viscosity estimate. Both pressure and viscosity models were developed using grey-box modeling with GA. Potential to relate model parameters on different grades of the same polymer (low density polyethylene) has been demonstrated. However, extension of the technique to materials exhibiting significantly different properties and to processing in more complex industrial extruders would result in larger models and loss of physical meaning. In this project, greater use of first-principle models is employed with the aim of identifying a simple model structure which, in combination with a mechanism to correct for over-simplifications in the model, can capture the viscosity dependence of a wide range of polymeric materials and different machines and dies.
Based on our previous work, we have further investigated the soft-sensor approach for real time monitoring of viscosity. The soft-sensor approach (See Figure 1) involves a finite impulse response prediction model with adaptable linear parameters for real-time prediction of the melt viscosity based on the process inputs; the model predictions is then used as an input of a feedback model with a simple fixed structure to predict the barrel pressure which can be measured online. Finally, the predicted pressure is compared to the measured value and the corresponding error is used as a feedback signal to correct the viscosity estimate. This novel feedback structure enables the online adaptability of the viscosity model in response to modelling errors and disturbances, hence producing reliable viscosity estimate. It has been shown that the resulting system is able to track changes in viscosity as a result of changes in process conditions or feed material/die/extruder change, and the error is within 1%.
In conclusion, the soft sensor technology investigated in this project enables tracking of the key performance targets of the process and facilitates identification and appropriate response to system disturbances. Using the error between the predicted and the measured barrel pressure as a feedback to correct the viscosity estimate which has made the real time monitoring of viscosity feasible and reliable. The soft sensor developed can greatly help to reduce the need for expensive measuring devices and to develop robust on-line system identification and real time control for different feed materials and operation conditions [J1].
Task A.4 Viscosity control
We have reviewed a number of nonlinear control methodologies and developed a model free fuzzy controller to minimize the variation in melt pressure and temperature, thus reducing the variation of viscosity. The experimental results of the developed controller on a Killion KTS single extruder confirm the efficacy of the proposed fuzzy controller over traditional PID controller [C11, T2].
Task B. Identification and Control of Process Instabilities
Task B.1 Identification and control of melting instabilities
We have developed a torque signal acquisition method, and set up and carried out thermal stability trials at both Queen’s University Belfast and University of Bradford for data analysis and modelling.
Inferential monitoring of screw load torque to predict process fluctuations
We have investigated (1) an inferential monitoring approach of the screw load torque signal in an extruder by monitoring the armature current, without involving a physical torque measurement. This represents a good alternative approach to extract actual screw load torque dynamics in real time as direct measurement or observation of the screw load torque is rather difficult in practice; (2) the torque signal was dominated by the solid friction in the extruder and as such is useful for online identification of solids conveying issues; (3) correlations between melt temperature fluctuations and screw load torque fluctuations. However, it has been observed that these signals are not strongly correlated. It seems that as the screw torque signal is dominated by the solids conveying torque it is not sensitive enough to identify unstable melting issues. Conversely, experimental observations showed that pressure measurements can provide some insight to melting fluctuations and hence monitoring of both pressure and torque can help to diagnose some of variations as either melting or solids conveying online in a process [J4, C5, C6].
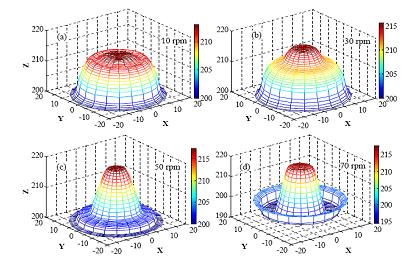
Investigation of melt temperature homogeneity in polymer extrusion
Thermal instability originating from the melting mechanism in polymer extrusion is a particular problem for many extrusion machines. Screw design has a major role in achieving thermal homogeneity, but optimisation of process settings to avoid such instabilities is increasingly important to achieve maximum efficiency and stability for a range of polymers on a fixed machine. Thermal instabilities and the onset of solid-bed break-up are difficult to model and predict, hence on-line identification of the effect of process settings on the onset of thermal instabilities is required. We have employed a thermocouple mesh technique originally developed by the University of Bradford [9] to measure the die melt temperature profile of a single screw extruder (See Figure 2). Very useful and detailed information was obtained from experiments with the thermocouple mesh in the evaluation of the effects of screw geometry, material properties and process settings on the process thermal homogeneity. It is clear that the process thermal homogeneity is significantly affected by all the conditions tested, i.e. screw geometry, process settings and material properties while the melt flow behaviour is highly variable over different processing situations. Moreover, it seems that these factors are highly correlated and this may cause the process to become highly unpredictable and variable in nature. Process settings and screw geometry show significant effects on the melt thermal homogeneity which may be due to the influence of these factors on process heat generation, material mixing, material residence time, etc. The same processing material shows considerably different thermal behaviours with different screw geometries and process settings. These observations emphasize the importance in selecting the screw and process settings appropriately for a particular material to avoid unnecessary thermal fluctuations which can cause severe problems to the product quality. As the processing behaviour may vary significantly throughout the process operation period, the continuous observation of the process thermal stability in real-time is invaluable to minimize any possible undesirable effects. Unfortunately, the detailed process monitoring techniques such as used in these experiments (i.e. thermocouple mesh) are not yet industrially available. Therefore, an attempt was made to predict the process fluctuations (including thermal homogeneity) from readily measureable process parameters to check the possibility of using them as powerful tools for industrial applications in predicting process stability [C3, J11].
Modelling and optimization of extruder output melt flow temperature profile
A novel static nonlinear polynomial model has been developed to predict the die melt temperature profile from readily measured process parameters. The model can predict the die melt temperature profile accurately over a wide operating range suggesting that this is a promising approach for determining melt flow thermal homogeneity compared to conventional point based temperature measurements. The model was used to identify the effects of individual processing parameters on the die melt flow homogeneity. Predictions from the proposed model are in agreement with previously reported experimental findings. Moreover, the model was used to identify the optimal process settings to minimise melt temperature variance across the melt flow with two different optimisation techniques. It has been shown that there is a potential to reduce melt temperature variations by between 2.9% and 33.3% over the 10 processing situations tested [J2, C5,C7].
The studies carried out in this research on optimising process thermal efficiency in polymer extrusion gave promising results and these results highlight the suitability of using such approaches to improve the productivity of industrial processes. In fact, there are number of ways to select the objective function for an optimisation problem and this should be selected based on the key process requirements. In this project, the achievement of the desired average melt temperature across the extruder output melt flow while minimizing temperature variance was considered and the objective function was selected on this basis. The results obtained with Differential Evolution techniques showed a reduction of melt temperature variance of up to 3.9% - 60.9% over the processing situations tested while achieving the desired average melt temperature across the melt flow. If the objective function was to set only to minimise the melt temperature variance, optimum process settings might be achieved with further reductions of melt temperature variance. These proposed methods for optimum process settings selection may enable an operating point with good thermal efficiency to be identified more quickly and in a logical way at a high screw speed (i.e. with a good energy efficiency), and hence design and control a polymer processing with less waste of material, energy and labour [J2].
Our main research findings in Task B.1 include
- The experimental evaluations suggested that the screw load torque was dominated by changes occurring in the solids conveying zone of the extruder and therefore process changes in other zones where molten material exists are less likely to be extractable from these torque dynamics [J4,C5,C6].
- The melt flow thermal homogeneity was found to be dependent on materials, process settings and machine geometry in a complex manner from a study carried out by measuring the extruder output melt flow temperature profiles under the different process settings, materials and screw geometries [J2].
- A novel methodology was proposed to model the extruder output melt flow temperature profile from readily measurable process parameters (i.e. screw speed and barrel set temperatures) and the predicted profiles showed good agreement with the experimentally measured profiles [J3,C5].
- The screw speed was identified as the most significant process variable on the level of the extruder output melt temperature and the temperature homogeny across the melt flow. Of the barrel zone temperatures, the metering zone temperature was identified as the most significant barrel zone temperature on the level of the extruder output melt temperature and the temperature homogeneity across the melt flow under the dynamic processing conditions despite the differences in materials, screw geometry and process settings [J3,C4].
- The selected optimum process parameters, with differential evolution technique, by using the static melt temperature profile prediction models gave reductions of the melt temperature variance of up to 3.1% - 60.9% while achieving the desired average melt temperature across the melt flow [J2].
- A novel soft sensor technique with a physical temperature feedback was introduced for real-time prediction of the extruder output melt flow temperature profile and the simulation results showed good performance by predicting the melt temperatures at the different radial locations across the melt flow. This was confirmed by the soft sensor’s feedback errors being less than ±5oC (i.e. less than ±2.25% from the full scale reading) over 129 minutes of prediction [J3].
Task B.2 Identification and control of feeding instabilities
The research described above shows that the torque signal was dominated by the solid friction in the extruder and as such is useful for online identification of solids conveying issues. However, to experimentally investigate the identification and control of solid conveying instabilities requires significant modification on the extruder machines, which was too ambitious for a PhD student to carry out within the limited time available.
Task C. Integration of sensor/control framework
We have explored energy constraints and developed a strategy for responding to process instabilities. We have investigated a model based fuzzy control approach [11,12] to maintain the die melt temperature variance across the melt flow while achieving the desired average die melt temperature. The developed controller determines the average melt temperature based on a radial temperature profile of the die melt flow rather than a point based measurement which is less accurate and quite common in practice. We have found that knowledge-based fuzzy rules provide good control capabilities to maintain the melt temperature homogeneity within desired limits by manipulating screw speed and barrel set temperatures in parallel. This provides a new method to operate extruders at high screw speeds whilst achieving both energy and thermal efficiencies in the same time [J2, C2]. We have further developed a fuzzy controller for the control of the polymer melt quality including viscosity, and it has been tested in laboratory experiments with confirmed efficacy [C11, T2].
4. Additional Achievements
Some additional outcomes that were not on the original list of objectives have been achieved:
- A nonlinear multivariate statistical approach based on neural networks has been developed for fault detection of nonlinear processes [J6 C10]. A Fast Recursive Algorithm [8] was employed to determine the optimal network topology, i.e. to identify the number of nodes, the location of centres, and the weights between the hidden layer and the output layer simultaneously for the radial basis function networks. In particular, we have proposed the use of nonlinear principal component analysis (PCA), which integrates principal curves and radial basis function (RBF) networks, to detect faults for nonlinear processes. To further solve the problem of the inclusion of a large number of variables in the RBF network input, we have further investigated a nonlinear dynamic PCA approach which employs an input selection scheme to pre-process and select only the most important time-lagged variables prior to building the RBF model. This helps in reducing the number of variables to be modelled and the related model complexity [C8]. The proposed methods have then been applied for fault diagnosis in polymer extrusion processes [J5,C9].
- We further investigated intelligent modelling and control methods using neural and fuzzy systems, and proposed novel algorithms for the construction of neural networks and fuzzy neural systems [ J7, J8, J10, C12, C13, C15], and nonlinear system modelling and classification [J9, C14]. The methods have also been applied to the modelling and control of injection stretch blow moulding [C16].
5. Research Impact, Benefits and Dissemination
We expect our research to have very considerable impact, in various ways, from stimulating further research inter-nationally, to the direct use of our novel algorithms and methodology in the polymer industry. The following represents only a few examples:
- Research in novel, inferential monitoring techniques and incorporation of on-line data analysis to achieve robust and adaptable control enables a much more holistic approach to optimisation of settings, disturbance rejection and self-tuning ability than previously attempted.
- Key technologies have been developed for addressing the poor energy efficiency and high volume of waste currently threatening the competitiveness of the UK polymer industry.
- The developments in process modelling and monitoring of key parameters for process optimisation and control will provide an ideal platform for achieving greater success in emerging complex processing applications. Related work by Dr. Marion McAfee during the period of the project identified a strong correlation between melt viscosity during processing and the degree of exfoliation of nanoclay in a polymer matrix [13]. Recent work has also identified that melt viscosity during processing is a good indicator of in-vitro degradation of bioresorbable polymers – a key performance characteristic for the development of temporary and drug-eluting medical devices [14]. The monitoring and control technologies developed in this project clearly show potential to accelerate the development of scalable manufacturing processes for nano-composite and bioresorbable products.
- Training of PhD and MEng students on advanced polymer control will help to increase the skills of the workforce to improve the competitiveness of UK polymer industry.
Considerable effort has been expended in effective dissemination of the research results. Mechanisms for dissemination have included:
- Publication of research results in refereed international journals, conference proceedings, PhD thesis and MEng final year project (see Section 8).
- Over 16 presentations at international conferences by the project team, targeting automatic control, system identification, fault detection and diagnosis, and polymer processing meetings. This includes IEEE Conference on Decision and Control and European Control Conference, International Federation of Automatic Control (IFAC) World Congress, Society of Plastics Engineers’ Annual Technical Conference (ANTEC), Polymer Processing Society Europe/Africa Regional Meeting.
- During this project period, the PI Prof Kang Li has been presented with the Young Author Best Paper Award at the 2011 International Conference on Modelling, Identification and Control (ICMIC 2011), held in Shanghai, China in June 2011, for the team work on modelling and control of polymer extrusion processes.
- To increase the international visibility of the advanced research on polymer control in the UK, Prof Kang Li has also been invited to give 2 research seminars on advanced polymer control in Tsinghua University and Shanghai University in China in 2010 and 2011. This follows an invited presentation from Dr. Marion McAfee on polymer processing monitoring and control at the US-Ireland Emerging Technologies Conference at University of Massachusetts Lowell in 2009.
This project has made considerable progress on developing an integrated framework for improved performance of extrusion processes through advances in process modelling, inferential measurement and a more intelligent approach to control of the process. Each of the topics has been intensively researched, including investigating novel grey-box modelling techniques capable of capturing the complex nature of interactions between process inputs and outputs of interest on industrial scale extruders and development of new mechanisms for on-line updating of models to reflect changes in material properties and processing conditions. A novel inferential measurement techniques for tracking melt viscosity and melt-temperature profile and identification of process instabilities has been proposed, and an advanced intelligent control strategy have been initiated to achieve the required product quality at maximum efficiency and respond appropriately to process faults. These new developments take into account the industrial application environment, and close collaboration with a leading research group and local industrial companies has enabled the direct application of the developed techniques to maximize the impact. Theoretic results have been published in high quality peer reviewed journals, such as Polymer Engineering and Science, Control Engineering Practice, IEEE Transactions on Fuzzy Systems, and Journal of Process Control, as well as leading conferences in the field of polymer engineering and control engineering. The publication record (11 international journal papers and 16 conference papers, together with 1 PhD thesis and 1 MEng final year project report), the award of international conference prize, and invited seminars have effectively demonstrated the high quality of research. We believe the research outcomes arising from this project present significant advances in the polymer extrusion control for energy and material saving and improvement of product quality.
7. List of publications arising from this research
Journal papers
J1. X. Liu, K. Li, M. McAfee, B.K. Nguyen, G. McNally, “Dynamic Grey-box Modeling for On-line Monitoring of Polymer Extrusion Viscosity’’, Polymer Engineering and Science, 2012, pp 1-10, DOI: 10.1002/pen.23080.
J2. C. Abeykoon-, K. Li, M. McAfee, P.J. Martin, Q. Niu, A. L. Kelly, J. Deng, “A new model based approach for the prediction and optimisation of thermal homogeneity in single screw extrusion”, Control Engineering Practice, Vol 19, No 8, 2011, pp 862-874.
J3. C. Abeykoon-, K. Li, P.J. Martin, A.L. Kelly, “Monitoring and Modelling of the Effects of Process Settings and Screw Geometry on Melt Pressure Generation in Polymer Extrusion”, International Journal of System Control and Information Processing (IJSCIP), ,in press, 2012
J4. C. Abeykoon-, M. McAfee, K. Li, P. J. Martin, A. L. Kelly, “The inferential monitoring of the screw disturbance torque to predict process fluctuations in polymer extrusion”, Journal of Materials Processing Technology, Vol 211, No 12, 2011, pp 1907-1918.
J5. X. Liu, K. Li, M. McAfee, Deng, J. “Application of nonlinear PCA for fault detection in polymer extrusion processes’’, Neural Computing and Applications, 2011, DOI: 10.1007/s00521-011-0581-y.
J6. X. Liu, K. Li, M. McAfee, G.W. Irwin, “Improved nonlinear PCA for process monitoring using support vector data description’’ Journal of Process Control, Vol.21, No.9, 2011, pp.1306-1317.
J7. Jing Deng, K. Li, George W. Irwin, Minrui Fei, "Two-stage RBF network construction based on particle swarm optimization", Transactions of the Institute of Measurement and Control, doi: 10.1177/0142331211403795, 2011.
J8. Jing Deng, K Li, George W. Irwin, "Locally regularised two-stage learning algorithm for RBF network centre selection", International Journal of Systems Science , DOI: 10.1080/00207721.2010.545490, pages 1157-1170, Volume 43, Issue 6, 2012.
J9. Jing Deng, K Li, George W. Irwin, "Fast automatic two-stage nonlinear model identification based on Extreme Learning Machine", Neurocomputing, 74 (16): 2422-2429, 2011, doi:10.1016/j.neucom.2010.11.035.
J10. Wanqing Zhao, K. Li, and George W. Irwin, "A New Gradient Descent Approach for Local Learning of Fuzzy Neural Models", IEEE Transactions on Fuzzy Systems, 2012, (DOI:10.1109/TFUZZ.2012.2200900, in press).
J11. C. Abeykoon-, P.J. Martin, A. L. Kelly, E.B. Brown, “A review and evaluation of melt temperature sensors for polymer extrusion”, Sensors and Actuators A: Physical, 2012,(DOI: 10.1016/j.sna.2012.04.026, in press).
Conference papers
C1. X. Liu, K. Li, M. McAfee, J. Deng "'Soft-sensor' for Real-time Monitoring of Melt Viscosity in Polymer Extrusion Process", 49th IEEE Conference on Decision and Control, December 15-17, 2010, Atlanta, Georgia USA,pp. 3469-3474.
C2. C. Abeykoon, K. Li, M. McAfee M, P.J. Martin and GW Irwin, ‘Extruder melt temperature control with fuzzy logic’, Proc. 18th IFAC World Congress, Bittanti, S and Cenedese A, Zampieri S (eds), ISBN: 978-3-902661-93-7, Milan, Italy, Aug 28- 2 Sept 2011, DOI: 10.3182/20110828-6-IT-1002.01576
C3. C. Abeykoon, K. Li, P. J. Martin, M. McAfee, “Modelling of Melt Pressure Development in Polymer Extrusion: Effects of Process Settings and Screw Geometry”, Proceedings of 2011 International Conference on Modelling, Identification and Control (ICMIC 2011), Shanghai, 26-29 June 2011, pp 180-185.
C4. C. Abeykoon, K. Li, M. McAfee, P. Martin, J. Deng, A.L. Kelly, "Modelling the effects of operating conditions on die melt temperature homogeneity in single screw extrusion" (2010), UKACC International Control Conference, Coventry, UK, pp 42-47
C5. C. Abeykoon, M. McAfee, K. Li, A. L. Kelly, E. C. Brown, “Investigation into monitoring and control of melt temperature homogeneity in single-screw extrusion”, ANTEC 2010, May 16-20, 2010, Orlando, Florida, pp:1799-1806.
C6. C. Abeykoon, M. McAfee, S. Thompson, K. Li, A.L. Kelly, E.C. Brown, “Investigation of torque fluctuations in extrusion through monitoring of motor variables”, Proceedings of the Polymer Processing Society Europe/Africa Regional Meeting ~ PPS-2009 ~ October 18-21, 2009 Larnaca (Cyprus).
C7. C. Abeykoon, K. Li, P. J. Martin, M. McAfee, J. Deng, A. L. Kelly, “Modelling the Effects of Operating Conditions on Die Melt Temperature Homogeneity in Single Screw Extrusion”, Lecture Notes in Computer Science, 2010, Volume 6329, Life System Modeling and Intelligent Computing, Pages 9-20. 2010 International Conference on Life System Modeling and Simulation & 2010 International Conference on Intelligent Computing for Sustainable Energy and Environment (LSMS & ICSEE 2010), September 17-20, 2010, Wuxi, China.
C8. X. Liu, K. Li, S. Li, M. Fei, “Input Selection for Dynamic Model of RBF Networks in Process Monitoring”, WCICA 2012, China (accepted).
C9. X. Liu, K. Li, M. McAfee, “Polymer Extrusion Process Monitoring Using Nonlinear Dynamic Model-based PCA”, UKACC International Conference on Control, Cardiff, UK, 3-5 September 2012, accepted.
C10. X. Liu, K. Li, M. McAfee, and J. Deng, “Improved Nonlinear PCA Based on RBF Networks and Principal Curves”, Lecture Notes in Computer Science 2010, Volume 6328, Life System Modeling and Intelligent Computing, Pages 7-15. 2010 International Conference on Life System Modeling and Simulation & 2010 International Conference on Intelligent Computing for Sustainable Energy and Environment (LSMS & ICSEE 2010), September 17-20, 2010, Wuxi, China.
C11. J. Deng, K. Li, E. Harkin-Jones, M. Fei, “Fuzzy logic based melt quality control of a single screw extruder”, The Fourth International Conference on Applied Energy (ICAE2012), Suzhou, July 5-8, 2012 (accepted).
C12. L. Zhang, K. Li, E. Bai, "A Novel Two-Stage Classical Gram-Schmidt Algorithm for Wavelet Network Construction", 16th IFAC Symposium on System Identification, SYSID 2012, Brussels, Belgium, July 11-13, 2012 (accepted).
C13. J. Deng, K. Li, G. W. Irwin, and M. Fei, " A fast forward RBF network construction with particle swarm optimization", Lecture Notes in Computer Science, 2010, Volume 6329, Life System Modeling and Intelligent Computing, Pages 40-48. 2010 International Conference on Life System Modeling and Simulation & 2010 International Conference on Intelligent Computing for Sustainable Energy and Environment (LSMS & ICSEE 2010), September 17-20, 2010, Wuxi, China.
C14. Jing Deng, K. Li, George W. Irwin, Robert F. Harrison, "A Fast Automatic Construction Algorithm for Kernel Fisher Discriminant Classifiers", 49th IEEE Conference on Decision and Control, December 15-17, 2010, Atlanta, Georgia USA, pp 2825-2830.
C15. Wanqing Zhao, K. Li, G. Irwin, "An Integrated Method for the Construction of Compact Fuzzy Neural Models", Lecture Notes in Computer Science, 2010, Volume 6215, Advanced Intelligent Computing Theories and Applications, Pages 102-109. 2010 International Conference on Intelligent Computing (ICIC2010), August 18-21, 2010, Changsha, Hunan, China.
C16. Jing Deng, Ziqi Yang, Kang Li, Gary Menary, 'Heuristically optimized RBF network for the modeling of sections weights in Injection Stretch Blow Moulding', UKACC International Conference on Control, Cardiff, UK, 3-5 September 2012, accepted.
Thesis and report
T1. C. Abeykoon, “Modelling and Control of Melt Temperature in Polymer Extrusion”, PhD thesis, Queen’s University Belfast, Sept 2011.
T2. D. McAuley, “ Polymer Extrusion Control”, MEng (Hons) Final Year Project Report, May 2012
8. Other references
1. Chiu, S.H. and Pong, S.H., “In-line viscosity control in an extrusion process with a fuzzy gain scheduled PID controller,” Journal of applied polymer science, vol. 74, pp.541-555, 1999.
2. M. McAfee and S. Thompson, “A novel approach to dynamic modelling of polymer extrusion for improved process control,” Proceedings of the Institution of Mechanical Engineers, Part I, Journal of Systems and Control, vol. 221, no. 4, pp. 617–628, 2007.
3. M.H. Iqbal, U. Sundararaj, and S.L. Shah, “New approach to develop dynamic gray box model for a plasticating twin-screw extruder,” Industrial & Engineering Chemistry Research, vol. 49, no. 2, pp. 648–657, 2010.
4. M.H. Iqbal, U. Sundararaj, and S.L. Shah, “Identification of transient responses of a plasticating twin screw extruder due to excitation in feed rate,” Polymer Engineering & Science, vol. 51, no. 3, pp. 482–491, 2011.
5. Li, K., Thompson, S., Duan, G.R., and Peng J.X., “A case study of fundamental grey-box modelling,” 15th IFAC World Congress on Automatic Control, Barcelona, Spain, 2002.
6. Li, K., Thompson, S., and Peng J.X., “Modelling and prediction of NOx emission in a coal-fired power generation plant,” Control Engineering Practice, vol. 12, pp.707-723, 2004.
7. Li, K. and Peng J.X., “System oriented neural networks - problem formulation, methodology and application,” International Journal of Pattern Recognition and Artificial Intelligence, vol. 20, pp. 143-158, 2006.
8. K. Li, J. Peng, and G. W. Irwin. “A fast nonlinear model identification method,” IEEE Transactions on Automatic Control, vol. 50, no. 8, pp.1211–1216, 2005.
9. E.C., Brown, A.L., Kelly, P.D., Coates, “Melt temperature field measurement in single screw extrusion using thermocouple meshes,” Review of Scientific Instruments, vol. 75, no.11, pp. 4742–4748, 2004.
10. E.C., Brown, A.L., Kelly, P.D., Coates, “Melt temperature homogeneity in single screw extrusion: effect of material type and screw geometry,” SPE ANTEC Tech. papers, pp.183–187, 2004.
11. M.H., Costin, P.A., Taylor, J.D., Wright, “On the dynamics and control of a plasticating extruder,” Polymer Engineering Science, vol. 22, no.17, pp.1095–1106, 1982.
12. A.M.F., Fileti, A.J.B., Antunes, F.V., Silva, “Experimental investigations on fuzzy logic for process control,” Control Engineering Practice, vol. 15, pp.1149–1160, 2007.
13. McAfee M., Chang W.C., ‘On-line optical monitoring of nanocomposite compounding’. ANTEC 2009, Society of Plastic Engineers, Chicago, 22-24 June 2009.
14. K. Paakinahoa, H. Heinoa,b, J. Väisänena, P. Törmäläb, M. Kellomäkia, “Effects of lactide monomer on the hydrolytic degradation of poly(lactide-co-glycolide) 85L/15G” Journal of the Mechanical Behaviour of Biomedical Materials, vol. 4, pp.1283-1290, 2011.