Engine Fault Diagnosis Using Data Modelling
Researchers: Prof. GW Irwin, Dr. G McCullough, Dr. X Wang, Dr. N McDowell and Dr U Kruger
Project Description
The regulations associated with current on-board diagnostic (OBD) systems demand very strict monitoring of engine performance at certain intervals. This monitoring entails the diagnosis of any fault which could cause the tailpipe emissions of carbon monoxide, unburned hydrocarbons and oxides of nitrogen to rise above legislated values. In the absence of durable and affordable sensors to monitor the concentrations of these gases on the vehicle, coupled with the extremely low emissions limits imposed by future legislation, complying with OBD requirements is an extremely challenging task.
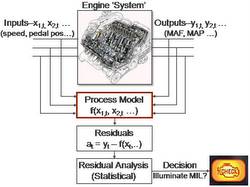
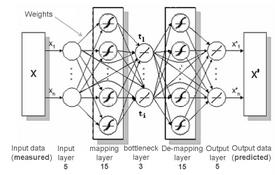
A multidisciplinary research team at QUB in the Virtual Engineering Centre, drawn from the Intelligent Systems and Control and the Internal Combustion Engines research groups, has pioneered a completely new approach to on-board diagnostic systems for internal combustion engines using multivariate statistical process control. In particular, an Auto-Associative Neural Network (Fig 2) implementation has proved effective in handling the inherent nonlinearities in the data modelling. This approach is capable of analysing the highly dynamic and non-linear signals provided by sensors currently available on production vehicles and it is envisaged that its performance will exceed existing OBD techniques.
For more information please contact
Prof. George Irwin (Intelligent Systems and Control)
Dr Geoff McCullough (Internal Combustion Engines)
External Lectures
Prof GW Irwin, ‘Automotive engine fault detection’, Invited lecture at EPSRC Winter School on Data Modelling, University of Sheffield, 21-25 January 2008.
Dr N McDowell, ‘Fault diagnosis for internal combustion engines - current and future techniques’, Invited lecture at 3rd IET Conference on Automotive Electronics, University of Warwick, Coventry, 28-29 June 2007.
Prof GW Irwin and Dr U Kruger,‘Intelligent use of data for condition monitoring and applications’, Invited Plenary, 2nd International Conference on Intelligent on Intelligent Computing (ICIC ’06), Kunming, Yunnan Province, China, August 2006.
Dr U Kruger, ‘Development and Application of nonlinear PCA for fault diagnosis in internal combustion engines’, Invited Speaker, Workshop on ‘Principal Manifolds for Data Cartography and Data Reduction’, Department of Mathematics, University of Leicester, 24-26 August 2006.
Achievements
- Innovative OBD technology proven experimentally on a Euro 3 engine
- Reduced complexity using semi-physical models for data pre-processing
- New identification cycle for non-linear, dynamic modelling
- Integration of the Local Approach for improved monitoring sensitivity
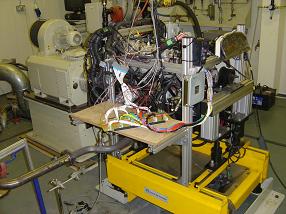
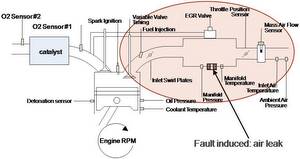
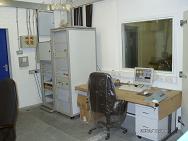
The researchers gratefully acknowledge the Engineering and Physical Sciences Research Council (EPSRC) for funding this project (EP/C005457/1).
Publications
Journals
- Wang X., Irwin G.W., McDowell N., McCullough G., and Kruger U., ‘Application of nonlinear dynamic PCA to automotive engine modeling and fault monitoring’, Control Engineering Practice, under review.
- McDowell N, McCullough G, Wang X, Kruger U, and Irwin GW, ‘Fault diagnostics for internal combustion engines – current and future techniques’, SAE Journal of Engines, selected as outstanding technical contribution to SAE 2007 World Congress (conference paper 6), to appear (March 2008).
- Wang, X, Kruger, U, Irwin, G W, McCullough G and N McDowell, ‘ Nonlinear PCA with the local approach for diesel engine fault detection and diagnosis’, IEEE Trans. Control Systems Technology , Vol. 16, No. 1, pp. 122-129, Jan 2008. DOI 10.1109/TCST.2007.899744.
Professional Journal
- McDowell, N, McCullough, G, Wang, X, Kruger, U and Irwin, G W, ‘Fault diagnosis for internal combustion engines’, Automation Technology in Practice, Oldenbourg, Volume 4, Issue 3, 2006, p19-26.
Conferences
- Wang X, Irwin GW, McCullough G, McDowell N and U Kruger, ‘Transient IC engine monitoring under temperature changes using an AANN’, Proc. 4th Int. Workshop on Artificial Neural Networks and Intelligent
Information Processing, Funchal, Madeira, Portugal, 14-15 May 2008, pp.
28-40 and in CD Rom Proc. 5th Int. Conf. on Informatics in Control,
Automation and Robotics (ICINCO).
- Wang X, Irwin GW, McCullough G, McDowell N, and Kruger U, ‘Nonlinear PCA for transient monitoring of an automotive engine’, accepted in UKACC International Control Conference, September 2-4, Manchester, UK, (September 2008).
- Wang, X, McDowell, N, Kruger, U, McCullough, G and Irwin GW, ‘Semi-physical neural network model in detecting engine transient faults using the local approach’, IFAC World Congress, Soeul, Korea, (July 2008)
- McDowell N, McCullough G, Wang X, Kruger U and Irwin GW, ‘Fault detection in internal combustion engines using a semi-physical neural network approach’, Proceedings ICE2007 – 8th International Conference on Engines for Automobiles, September 16-20, Capri, Italy, Paper 2007-24-0050, (2007).
- McDowell N, Wang X, McCullough G, Kruger U and Irwin GW, ‘Application of Auto-associative Neural Networks to Transient Fault Detection in an IC Engine’, Proceedings ASME Internal Combustion Engine Division, Fall Technical Conference, Charleston, South Carolina, USA, Oct. 14-17, (2007), Paper No. ICEF2007-1728.
- McDowell N, McCullough G, Wang X, Kruger U, and Irwin GW, ‘Fault diagnostics for internal combustion engines – current and future techniques’, SAE 2007 World Congress, April 16-19, Detroit, Michigan, USA, (2007).
- Wang X, Kruger U, Irwin GW, McDowell N, and McCullough G, ‘Nonlinear PCA for Process Monitoring Using the Local Approach’, Proceedings 6th IFAC Symposium on Fault Detection, Supervision and Safety of Technical Processes, pp. 115-120, August 30 - September 1, Beijing, P.R. China, (2006).